Drone following instructions
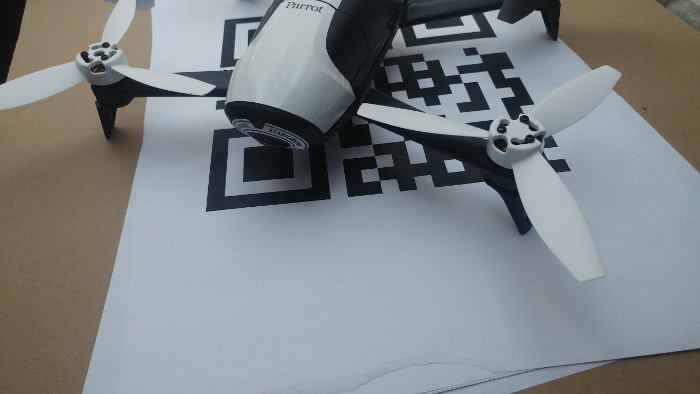
Reading instructions from QR codes and executing them using android application Intro Recently I got an opportunity to build a drone prototype controlled by Android device. Firstly I had to choose the best candidate. The requirements were: small size and SDK with a video streaming. After some research I've decided that the Bebop 2 from Parrot would be the best choice. Parrot is one of the few companies that has an open SDK for developers. Recently they have released the 3rd version of their SDK. The first step led to try the android application example . This example covers almost every basic feature: connecting to drone, moving around, making picture in high quality and accessing the drone's media. One of the steps for the prototype would be autonomous landing onto a pattern. I've done some research about the existing solutions and found this paper that describes the theory behind the landing. So I've decided to create an android application that navigates